In today’s data-driven world, understanding metrics like 74.09, 0.23, and 5-32.32 can transform how businesses and data scientists approach decision-making, whether in digital marketing, predictive modelling, or performance analysis.
Though seemingly arbitrary, these figures encapsulate crucial data analysis techniques and model performance metrics that provide a window into the effectiveness and accuracy of models.
This article dives into the statistical insights these numbers reveal, exploring their application across fields and unpacking how to interpret and use them to evaluate success, compare model performance scores, and improve model accuracy.
Implementing the Dynamic Digital Marketing Concept of 74.09 0.23 5-32.32
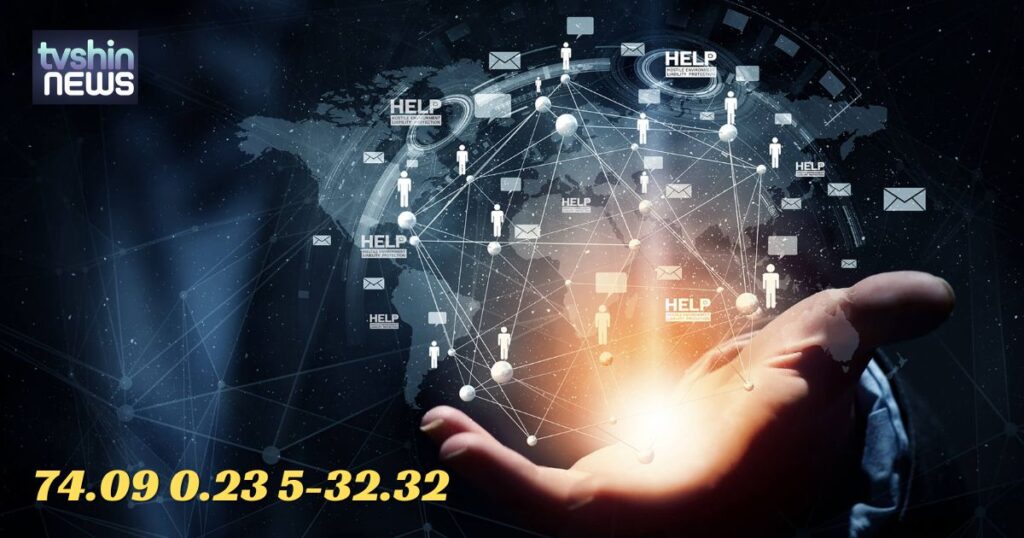
What Does 74.09 0.23 5-32.32 Mean in Digital Marketing?
Digital marketing relies on precise metrics to target audiences effectively, optimize content strategies, and monitor real-time campaign performance.
When we talk about 74.09, 0.23, and 5-32.32 in this field, each number can be interpreted to represent a valuable Key Performance Indicator (KPI):
- 74.09 – This could represent an engagement rate or customer retention metric, indicating how well a campaign resonates with its audience.
- 0.23 – Often seen as an error margin or adjustment factor, this value is crucial in fine-tuning targeting, ensuring minimal advertising waste.
- 5-32.32 – This range could signify conversion rates or revenue impact, showing the effectiveness of campaigns on business performance.
Incorporating these figures can provide statistical insights into which campaigns connect with customers and which need revision. For instance, analyzing data accuracy through performance benchmarks ensures the strategies align with organizational goals.
Steps to Apply the 74.09 0.23 5-32.32 Concept in Marketing
To make the most of these numbers, digital marketers should focus on the following:
- Audience Targeting with 0.23: Use this figure’s precision to create hyper-targeted campaigns that reach the ideal demographic, increasing engagement rates and minimizing costs.
- Content Optimization via 74.09: When engagement rates approach 74.09%, marketers can assume the content resonates well, possibly boosting reach organically.
- Conversion Tracking within 5-32.32: Marketers gain a clear picture of ROI by monitoring conversion rates in this range and can quickly adjust budgets to emphasize high-performing campaigns.
Integrating quantitative analysis ensures data-driven decision-making. Real-time analytics tools, such as Google Analytics or SEMrush, capture these metrics effectively, allowing for adaptive digital marketing strategies that respond to changes in audience behaviour.
Interpreting the Significance of 74.09 0.23 5-32.32 in Business Performance Analysis
Breaking Down Each Metric for Business Insights
In business, metrics like 74.09, 0.23, and 5-32.32 can provide detailed insights into financial performance, customer satisfaction, and operational efficiency.
- 74.09% – This could signify customer retention rates or satisfaction scores. A high retention rate indicates strong customer loyalty, which is essential for long-term success.
- 0.23 – Often used as a precision factor, a 0.23 value could represent an acceptable error margin, helping businesses avoid costly miscalculations in financial forecasts.
- 5-32.32 – This might represent a range of profit margins or revenue growth, helping businesses benchmark performance against industry standards.
Applying Metrics to Drive Business Decisions
Understanding and applying these numbers to business performance can pinpoint areas of strength or needed improvements. For example:
- Customer Satisfaction Analysis: If 74.09% of surveyed customers are satisfied, it may indicate a robust support system. Low scores, however, suggest opportunities for enhancing customer experience.
- Revenue Growth Forecasting: Using the 5-32.32 range as a projection for expected annual growth provides a tangible benchmark.
- Cost Analysis with 0.23 Error Margins: Maintaining this level of precision in forecasting protects against over-expenditures.
Historical performance analysis is another critical application here, allowing businesses to examine long-term trends and measure the effectiveness of strategies over time.
Essential Tools for Enhancing Your Website’s Performance
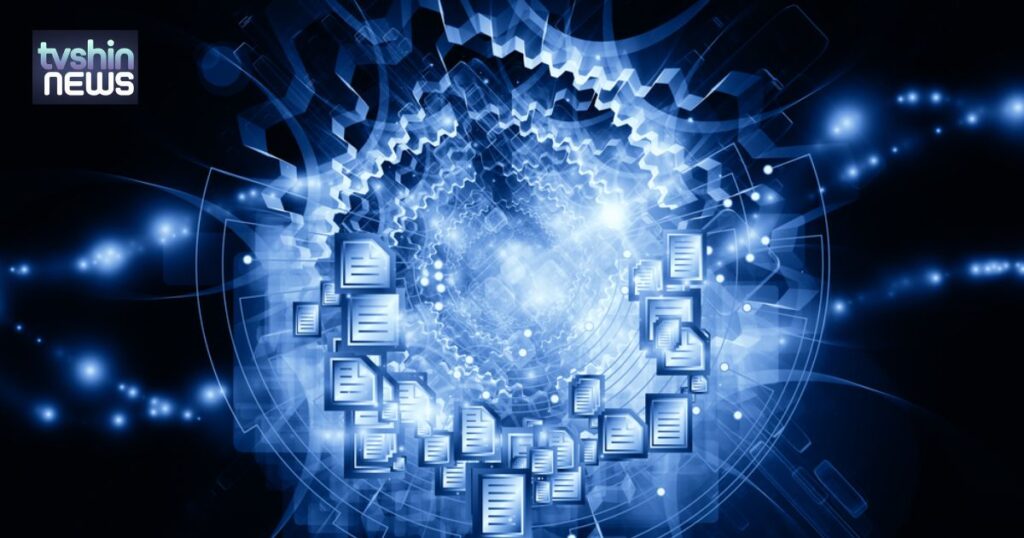
SEO and Analytics Tools to Capture Key Metrics
Several tools help capture and interpret performance metrics effectively for websites that maximize reach and conversions.
These tools can reveal the statistical relevance of model scores like 74.09 and 0.23, offering insights for website optimization.
- Google Analytics – Tracks engagement metrics, allowing marketers to measure bounce rates, session duration, and conversion tracking.
- SEMrush and Moz – Both tools focus on SEO health, providing data analysis techniques that guide traffic acquisition strategies.
- Heatmaps (e.g., Crazy Egg) – Offer visual insights into user interactions, supporting content optimization for better engagement rates.
Affordable Tools for Small Businesses
Budget-conscious businesses can rely on Google Search Console for SEO insights or Ubersuggest for cost-effective keyword research.
These tools ensure small businesses can still analyze data-driven performance without heavy investments.
Real-Time Feedback for Campaign Optimization
Setting up KPIs and real-time feedback dashboards to track metrics like traffic, bounce rates, and conversions enables dynamic response to market changes.
For example, performance benchmarks for models can highlight pages needing optimization, enhancing site user experience and engagement.
Analyzing Data: Insights from 74.09 0.23 5-32.32
Interpreting metrics like these requires context to extract actionable insights. For instance, 74.09 as a performance metric might measure engagement in social media analytics, while 0.23 could represent model precision in predictive modelling.
Frameworks for Data Analysis
To analyze these metrics, applying frameworks like descriptive and inferential statistics is essential:
- Descriptive Analysis: Summarizes data, showing averages and trends.
- Inferential Analysis: This helps predict future performance, which is crucial for predictive modelling.
Real-World Examples and Case Studies
Case studies in fields like e-commerce reveal how data accuracy in conversion tracking (e.g., 74.09% as an engagement rate) significantly impacts strategy.
Such quantitative analysis not only refines business tactics but also enhances competitive advantage.
Measuring the Effectiveness of Your 74.09, 0.23, and 5-32.32 Strategies
Key Performance Indicators (KPIs) to Monitor
Monitoring KPIs tied to the 74.09 0.23 5-32.32 concept offers an ongoing gauge of campaign or model performance:
- Organic Traffic – Indicates brand visibility and engagement, correlating with metrics like 74.09.
- Conversion Rates – Reflect effectiveness in achieving goals, with 5-32.32 representing successful conversion ranges.
- Bounce Rate and Session Duration – Reveal user engagement, helping to understand if content resonates with visitors.
Analyzing and Optimizing for Better Results
Analyzing these KPIs helps identify improvement areas. Adaptive strategies can refine marketing campaigns based on these insights, driving enhanced ROI and audience engagement.
Embracing Adaptability in Digital Marketing Strategies
Why Adaptability Matters
In the evolving digital landscape, flexibility is critical to leveraging data-driven insights. A static approach limits success, especially when metrics reveal real-time trends or unexpected shifts in audience behaviour.
Techniques to Stay Agile
- A/B Testing: Continuously experiment to discover optimal strategies.
- Feedback Loops: Set up systems for immediate audience feedback.
- Data-Driven Adjustments: Analyze dataset metrics interpretation regularly to respond quickly.
By adapting based on these techniques, marketers can ensure sustained relevance and competitive edge in their campaigns.
Analyzing Machine Learning Model Performance: Insights from 74.09, 0.23, and 5-32.32
Understanding Model Accuracy and Error Margins
Machine learning requires precision to generate actionable insights. 74.09 could denote model accuracy or recall rate, while 0.23 might reflect an acceptable error margin.
- Accuracy (74.09%): Indicates model success in correctly predicting outcomes, with high percentages translating to reliable models.
- Error Rate (0.23): Minimizing error is crucial, especially in high-stakes applications like healthcare or finance.
- Parameter Tuning (5-32.32 Range): Adjusting model parameters within this range can enhance predictive modelling outcomes.
Techniques to Improve Model Performance
Techniques for improving machine learning model scores and refining performance benchmarks include:
- Cross-validation – Enhances accuracy by testing models on multiple subsets of data.
- Regularization – Reduces overfitting, ensuring models generalize well to new data.
- Hyperparameter Optimization – Fine-tunes model parameters, improving precision and recall.
Exploring 74.09 0.23 5-32.32 for Product Development and Market Fit
Product development relies heavily on market research and consumer feedback to ensure new offerings meet market demands.
Metrics like 74.09, 0.23, and 5-32.32 can provide valuable insights into a market fit, guiding teams toward creating products that fulfill customer needs and are highly likely to succeed.
For instance, 74.09% could serve as a customer satisfaction benchmark, helping teams gauge the appeal of a product prototype before full-scale production. 0.23 could indicate acceptable feedback variation, allowing for constructive criticism without skewing the overall product quality assessment.
The 5-32.32 range might reflect expected adoption rates in different demographics, guiding product launch strategies and resource allocation. By incorporating these metrics into quantitative analysis, product teams can make data-informed decisions that improve market fit and customer satisfaction.
Utilizing 74.09 0.23 5-32.32 in Financial Forecasting Models
Financial forecasting demands precision, as even minor prediction errors can result in significant economic losses.
Metrics like 74.09, 0.23, and 5-32.32 help analysts and financial planners validate forecast accuracy, improve error margins, and ensure reliable financial projections.
74.09 could indicate a confidence level in forecasting models, suggesting that predictions meet a particular threshold of reliability. Meanwhile, 0.23 may serve as a tolerance for error, helping analysts assess acceptable risk in projected outcomes.
The range of 5-32.32 can represent growth or revenue estimate fluctuations, which is critical for planning and budgeting. By applying these metrics, financial teams can make informed decisions about investments, budgeting, and resource allocation, ultimately reducing financial risk.
Building Robust Model Evaluation Techniques with 74.09, 0.23, and 5-32.32 Metrics
Model evaluation is a core component of data science, ensuring models perform effectively across varied datasets.
Metrics like 74.09, 0.23, and 5-32.32 can be benchmarks in evaluating models’ accuracy, precision, and robustness, especially in fields where reliable predictions are essential.
For example, 74.09% could represent an accuracy threshold, where models that meet or exceed this value are considered successful in classification tasks. 0.23 may signify an acceptable error rate, a standard measure in industries where data discrepancies impact decision-making.
Meanwhile, 5-32.32 could indicate the range of acceptable deviations in predictive outputs, allowing data scientists to set realistic expectations. By using these metrics, teams can compare model performance scores objectively and refine models until they achieve desired performance levels.
Enhancing Customer Segmentation with 74.09, 0.23, and 5-32.32 Metrics
Customer segmentation, the process of dividing a more extensive audience into distinct groups based on behaviour, demographics, or preferences, is essential for targeted marketing.
Metrics like 74.09, 0.23, and 5-32.32 can be valuable in refining segmentation models, ensuring each segment receives messaging and offers that resonate.
Using 74.09 as a benchmark for customer lifetime value (CLV) can help identify high-value customers, focusing resources on retaining these profitable segments. 0.23 might serve as a precision rate for segment classification, guiding marketers on the accuracy of their segmentation.
Additionally, the 5-32.32 range can represent variations in buying behaviour or engagement levels across segments, highlighting differences marketers can leverage in their strategies. These metrics make segmentation more data-driven and precise, leading to higher ROI and more effective customer targeting.
Leveraging 74.09 0.23 5-32.32 for Predictive Analytics in Marketing
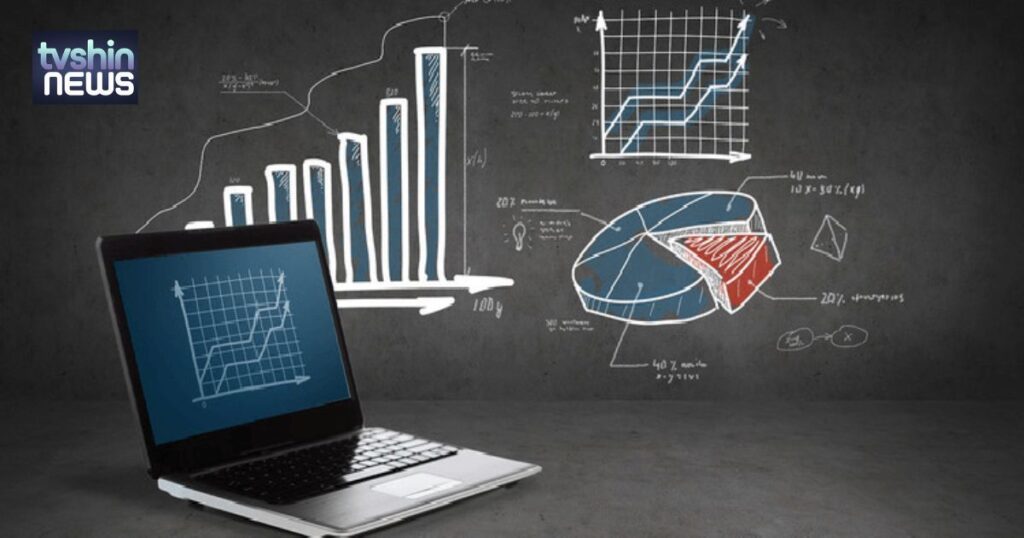
Predictive analytics enables marketers to anticipate trends, customer behaviours, and engagement, improving how they craft future campaigns.
Marketing teams can enhance their forecast accuracy and campaign targeting by incorporating metrics like 74.09, 0.23, and 5-32.32 into predictive models.
For instance, 74.09% could serve as a threshold indicating customer engagement or satisfaction levels. Campaigns will resonate well with the target audience when engagement exceeds this number.
Meanwhile, 0.23 could represent an acceptable margin for prediction error, allowing marketers to assess the statistical relevance of model scores and make informed adjustments without compromising effectiveness.
The range of 5-32.32 might signify expected variations in predicted conversion rates, which can guide budget allocation and audience segmentation. Marketers leveraging these insights can deliver more personalized, targeted experiences that enhance engagement and drive conversions.
Conclusion
74.09 0.23 5-32.32 represent more than mere numbers; they encapsulate vital insights into data analysis, business performance, and machine learning evaluation. Businesses can make data-informed decisions, refine marketing strategies, and optimize machine learning models by interpreting these metrics.
Using these metrics as performance benchmarks will drive better outcomes and foster adaptability in a competitive digital landscape. Embrace these data insights and let them guide you toward enhanced performance, agility, and strategic success.